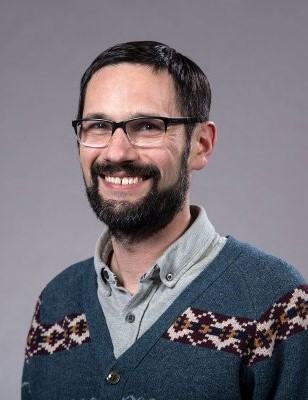
Abstract:
Impactful winter precipitation events are often heavily controlled by mesoscale airflow dynamics and small-scale physical processes. Consequently, effective numerical weather prediction (NWP) models for these events need to have high resolution and accurate representation of key parameterized processes. Furthermore, effective probabilistic NWP for these events requires the use of ensembles that account for uncertainty associated with initial/boundary conditions and parameterized physics.This talk will cover some of our recent work aimed at improving mesoscale NWP of impactful winter precipitation events using (1) stochastic physics parameterizations and (2) field project observations.
One way to represent the impact of physics scheme uncertainty in high-resolution ensemble forecasts is using stochastic parameter perturbation (SPP) to vary individual uncertain parameters within physics schemes. We are evaluating and improving the utility of SPP for ensemble forecasts of lake-effect snow and orographic precipitation, with a focus on near-surface turbulence and microphysical parameterizations. Case study results reveal that, especially for lake-effect snow, SPP can effectively improve ensemble spread, in a physically meaningful manner, while maintaining ensemble-mean performance.
The measurements of winter storms needed for detailed evaluation of high-resolution NWP are often unavailable from operational networks. We are leveraging unique observations from field campaigns to evaluate the performance of mesoscale NWP and target improvements. Observations from the 2022 Winter Precipitation Type Research Multi-scale Experiment (WINTRE-MIX) reveal large biases in precipitation type forecasts from operational mesoscale NWP. As an example, we document how forecasts of the transition between freezing rain and ice pellets are highly sensitive the representation of boundary layer turbulence and secondary ice crystal formation.